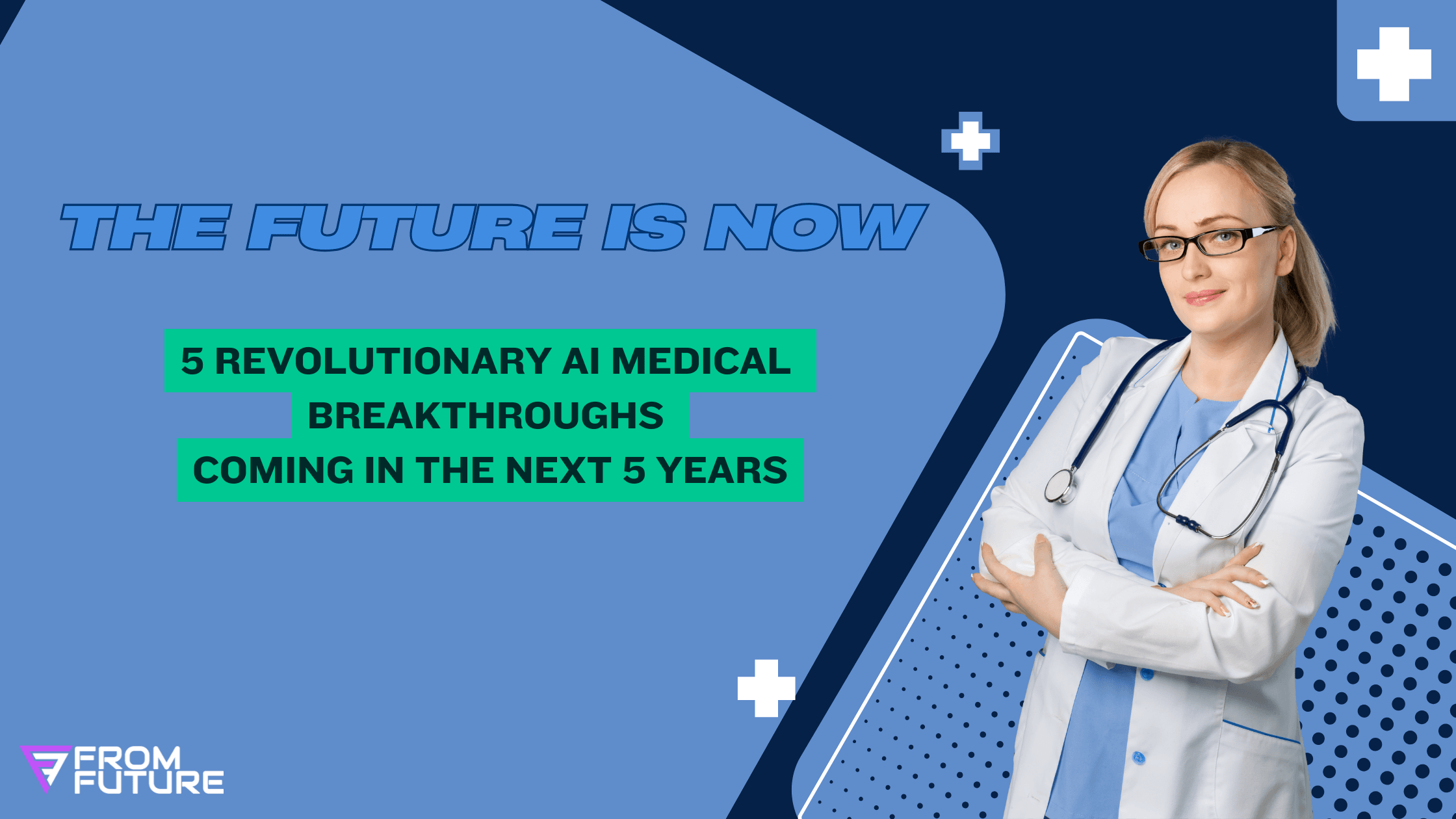
The Future is Now: 5 Revolutionary AI Medical Breakthroughs Coming in the Next 5 Years
Introduction: The Rapid Evolution of AI in Healthcare
Healthcare stands at the precipice of a technological revolution. Artificial intelligence (AI), once confined to the realm of science fiction, has emerged as a transformative force in medicine, promising to reshape how we diagnose, treat, and manage diseases. The complexity and rise of data in healthcare has created the perfect environment for AI to flourish, with algorithms now capable of analyzing vast datasets at speeds and accuracies that surpass human capabilities.
In recent years, we've witnessed the initial integration of AI into various aspects of healthcare—from diagnostic tools that detect cancerous lesions to virtual assistants that help manage patient data. However, what we've seen so far merely scratches the surface of AI's potential. The next five years promise to usher in breakthroughs that will fundamentally transform medical practice and patient care.
Why the Next 5 Years Will Be Transformative
According to research published in Future Healthcare Journal, "we expect to see limited use of AI in clinical practice within 5 years and more extensive use within 10." This timeline isn't arbitrary—it reflects the convergence of several critical factors: exponential growth in computing power, unprecedented access to healthcare data, advances in machine learning algorithms, and increasing acceptance of AI tools among healthcare professionals.
The COVID-19 pandemic has further accelerated this timeline, highlighting the need for innovative solutions to healthcare challenges and catalyzing digital transformation across the industry. As regulatory frameworks adapt and implementation barriers are addressed, we stand on the cusp of witnessing revolutionary changes in how healthcare is delivered.
This article explores five groundbreaking areas where AI is poised to make significant impacts in medicine over the next five years, examining both the immense potential and the challenges that lie ahead. From precision diagnostics to personalized treatment plans, the future of healthcare is being rewritten by intelligent algorithms—and that future is arriving faster than many anticipated.
AI Revolution in Medical Diagnostics
AI-Powered Medical Imaging: Seeing Beyond Human Capability
The field of medical imaging stands at the forefront of AI's impact on healthcare. Traditional image interpretation relies heavily on the human eye and expertise of radiologists, but AI systems are now demonstrating capabilities that extend beyond human perception. Deep learning algorithms, particularly deep convolutional neural networks (DCNNs), are revolutionizing how medical images are analyzed and interpreted.
According to a recent review of AI/ML-based medical devices approved in the USA and Europe from 2015-2020, more than half of all approved AI medical devices were for radiological use. This trend is accelerating as studies consistently demonstrate AI's ability to meet or exceed the performance of human experts in image-based diagnoses across multiple specialties.
What makes these systems particularly powerful is their ability to detect "radiomics" – clinically relevant features in imaging data that are imperceptible to the human eye. This capability allows for earlier detection of disease, more accurate diagnoses, and ultimately better patient outcomes. As one researcher noted in the journal BMC Medical Education, "ML, in short, can assist in decision-making, manage workflow, and automate tasks in a timely and cost-effective manner."
Early Cancer Detection: How AI is Saving Lives
Perhaps nowhere is AI's diagnostic potential more evident than in cancer detection. Multiple studies have demonstrated AI's remarkable accuracy in identifying various forms of cancer, often at earlier stages than human clinicians can achieve.
In breast cancer screening, a groundbreaking study in the UK showed that utilizing an AI system to interpret mammograms resulted in an absolute reduction in false positives and false negatives by 5.7% and 9.4%, respectively. Another study conducted in South Korea found that AI-based diagnosis was significantly more sensitive in detecting breast cancer with mass compared to radiologists (90% vs. 78%), and substantially better at identifying early breast cancer (91% vs. 74%).
Dermatology has seen similar advances. Deep learning algorithms have been trained to classify skin lesions with accuracy comparable to board-certified dermatologists. In a landmark study published in Nature, researchers demonstrated that a deep neural network could classify skin cancer with a level of competence comparable to dermatologists, requiring only a fraction of the training time compared to physicians who spend years in medical school and decades gaining clinical experience.
These breakthroughs aren't just academic achievements—they represent real potential to save lives through earlier detection and more accurate diagnosis of deadly diseases.
Revolutionizing Ophthalmology with AI Screening
Diabetic retinopathy, a leading cause of preventable vision loss worldwide, presents a significant public health challenge. With the growing prevalence of diabetes globally and limited resources for eye care, AI offers a promising solution for widespread screening.
Research studies on automated AI algorithms for diabetic retinopathy conducted in the USA, Singapore, Thailand, and India have demonstrated robust diagnostic performance and cost-effectiveness. These systems can analyze retinal images to detect signs of diabetic retinopathy with high sensitivity and specificity, enabling early intervention before vision loss occurs.
The FDA's approval of the first autonomous AI diagnostic system for diabetic retinopathy in 2018 marked a significant milestone. This system doesn't just assist ophthalmologists—it can make diagnostic decisions independently, expanding access to screening in areas with limited specialist availability.
AI in Cardiology and Respiratory Medicine
Cardiovascular and respiratory diseases remain leading causes of mortality worldwide, making accurate and timely diagnosis crucial. AI is making significant inroads in these fields as well.
In cardiology, deep learning algorithms have demonstrated the ability to diagnose heart attacks with performance comparable to cardiologists. More impressively, AI systems can now predict new-onset atrial fibrillation from standard ECG data, potentially identifying patients at risk before they experience symptoms. As noted in a 2021 study published in Circulation, these algorithms can "help identify those at risk of atrial fibrillation–related stroke," enabling preventive interventions.
For respiratory conditions, AI has shown remarkable capabilities in detecting pneumonia from chest radiography. One study found that AI systems achieved 96% sensitivity in pneumonia detection, compared to just 50% for radiologists. While the specificity was somewhat lower (64% for AI vs. 73% for radiologists), the significantly higher sensitivity means fewer cases go undetected.
As these diagnostic tools continue to mature over the next five years, we can expect to see their integration into standard clinical workflows, providing clinicians with powerful decision support and improving diagnostic accuracy across multiple medical specialties.
Transforming Drug Discovery and Treatment with AI
Accelerating Drug Development Timelines
The pharmaceutical industry faces enormous challenges in bringing new drugs to market. Traditional drug development is notoriously time-consuming and expensive, with the average new medication taking 10-15 years to develop at a cost of over $2.5 billion. Artificial intelligence is poised to dramatically transform this landscape over the next five years.
AI will drive significant improvement in clinical trial design and optimization of drug manufacturing processes. As noted in a recent ScienceDirect article, "any combinatorial optimization process in healthcare could be replaced by AI." We're already seeing the beginnings of this transformation with groundbreaking AI systems like DeepMind's AlphaFold, which has revolutionized protein structure prediction—a critical component of drug discovery.
By analyzing vast datasets of molecular structures, chemical properties, and biological interactions, AI algorithms can identify promising drug candidates far more efficiently than traditional methods. This capability not only accelerates the initial discovery phase but also helps predict which compounds are most likely to succeed in clinical trials, potentially saving years of development time and billions in research costs.
Precision Therapeutics: Tailoring Treatments to Individual Patients
The concept of "one-size-fits-all" medicine is rapidly becoming obsolete. AI is enabling a shift toward precision therapeutics—treatments tailored to individual patients based on their unique genetic makeup, environment, and lifestyle factors.
With better access to multimodal data (genomic, proteomic, glycomic, metabolomic, and bioinformatic), AI systems can handle far more systematic complexity than human researchers alone. This capability allows for more accurate predictions about which therapeutic agents will be effective for specific patients and which might cause adverse reactions.
Particularly promising is AI's ability to anticipate adverse drug effects, which have often thwarted the development of otherwise effective medications at costly late stages in the development process. By identifying potential safety issues earlier, AI can help pharmaceutical companies focus resources on the most promising candidates, ultimately democratizing access to novel advanced therapies at lower costs.
The Promise of AI in Immunomics and Synthetic Biology
Some of the most exciting breakthroughs in the next five years will come from the application of AI to immunomics and synthetic biology. Through the analysis of multimodal datasets, researchers are gaining unprecedented insights into the cellular basis of disease and the clustering of diseases and patient populations.
This deeper understanding enables more targeted preventive strategies and treatment approaches. The impact will be particularly revolutionary for cancer, neurological conditions, and rare diseases, where traditional approaches have often fallen short.
In the field of synthetic biology, AI is helping researchers design and optimize biological systems for therapeutic purposes. The combination of CRISPR gene editing technology with AI-driven design tools is opening new frontiers in personalized cancer therapies and treatments for genetic disorders.
According to research published in BMC Medical Education, these advances will "transform the way we understand, discover and affect biology," improving the efficiency of therapeutic development and ultimately providing more effective treatments for conditions that currently have limited options.
AI-Enabled Precision Medicine
From One-Size-Fits-All to Personalized Healthcare
Healthcare is undergoing a fundamental paradigm shift—moving away from the traditional one-size-fits-all approach toward a preventative, personalized, data-driven model of disease management. At the heart of this transformation is artificial intelligence, which enables the analysis of complex, multidimensional patient data at a scale and speed impossible for human clinicians alone.
"AI is perhaps the most transformational technology of our time, and healthcare is perhaps AI's most pressing application," notes Microsoft CEO Satya Nadella. This sentiment reflects the growing recognition that AI-enabled precision medicine represents the future of healthcare delivery.
In the next five years, we'll witness healthcare systems increasingly leveraging AI to achieve their "quadruple aim" by democratizing and standardizing a future of connected and AI-augmented care. This shift promises improved population health, enhanced patient experience, better caregiver experience, and reduced costs of care—goals that have long seemed at odds with one another.
Digital Twins: Testing Treatments in Virtual Environments
One of the most promising applications of AI in precision medicine is the development of "digital twins"—virtual models of individual patients that incorporate their unique biological, physiological, and genetic characteristics. These computational models allow clinicians to simulate how a patient might respond to different treatments before administering them in real life.
In the longer term, healthcare professionals will leverage AI in augmenting the care they provide, allowing them to deliver safer, standardized, and more effective treatment. Clinicians could use an "AI digital consult" to examine digital twin models of their patients, enabling them to "test" the effectiveness, safety, and experience of an intervention (such as a cancer drug) in the digital environment prior to delivering it to the patient in the real world.
This approach not only improves treatment efficacy but also reduces the risk of adverse effects, as potential complications can be identified and addressed in the virtual environment first. While full implementation of digital twin technology may extend beyond the five-year horizon, we'll see significant progress in this direction as the underlying AI models and data integration capabilities continue to mature.
Integrating Multi-Omic Data for Comprehensive Patient Profiles
The power of precision medicine lies in its ability to integrate diverse types of patient data to form a comprehensive picture of an individual's health. In the coming years, AI systems will increasingly combine genomic, radiomic, proteomic, clinical, and immunohistoric data—what some researchers call "holomics"—to develop highly personalized treatment approaches.
This integration of multi-omic data will be particularly transformative for complex conditions like cancer, where treatment response can vary dramatically between patients with seemingly similar disease presentations. By analyzing patterns across these multiple data types, AI algorithms can identify subtle biomarkers and disease signatures that predict treatment response with unprecedented accuracy.
According to research published in ScienceDirect, "In the medium term, we propose that there will be significant progress in the development of powerful algorithms that are efficient, able to use unlabelled data, and can combine disparate structured and unstructured data including imaging, electronic health data, multi-omic, behavioural and pharmacological data."
The result will be a healthcare system that achieves improved patient outcomes through AI-augmented healthcare and connected care, delivering a more cost-effective service that's personalized to each individual's unique biological profile.
Connected Care and Remote Monitoring
AI-Powered Virtual Assistants and Telehealth
The healthcare landscape is rapidly expanding beyond the confines of traditional clinical settings. In the next five years, AI-powered virtual assistants and telehealth solutions will become increasingly sophisticated and widespread, fundamentally changing how patients interact with healthcare systems.
Virtual assistants powered by natural language processing (NLP) are already being deployed to handle routine patient communications, appointment scheduling, and basic health inquiries. As these systems evolve, they'll become more capable of understanding context, recognizing emotional cues, and providing personalized health guidance.
Research published in Future Healthcare Journal indicates that "speech and text recognition are already employed for tasks like patient communication and capture of clinical notes, and their usage will increase." These technologies not only improve efficiency but also enhance accessibility, allowing patients to engage with healthcare systems on their own terms and schedules.
Telehealth platforms, which saw explosive growth during the COVID-19 pandemic, will become increasingly AI-augmented. Intelligent systems will help prioritize cases, suggest relevant questions for providers to ask, and even assist with preliminary diagnoses based on patient-reported symptoms and medical history. This evolution will make virtual care more effective and expand its applicability to a wider range of medical conditions.
Wearable Technology and Continuous Health Monitoring
The integration of AI with wearable devices represents one of the most promising developments in healthcare monitoring. From smartwatches that detect atrial fibrillation to continuous glucose monitors that predict diabetic events before they occur, AI-powered wearables are transforming how we track and manage health conditions.
The application of artificially intelligent systems in healthcare for use by the general public is expanding rapidly. The FDA's approval of devices like AliveCor's Kardiaband and Apple's smartwatch for detecting atrial fibrillation marked the beginning of a new era in consumer health technology. These devices represent "a first step toward empowering people to collect personal health data, and enable rapid interventions from the patient's medical support teams."
Over the next five years, we'll see significant advances in both the sophistication and accessibility of these technologies. Wearable sensors will become more accurate, less obtrusive, and capable of monitoring a wider range of physiological parameters. Meanwhile, the AI algorithms analyzing this data will grow increasingly adept at identifying subtle patterns that precede health events, enabling truly preventive interventions.
Extending Healthcare Beyond Hospital Walls
Perhaps the most transformative aspect of AI-powered connected care is its ability to extend high-quality healthcare beyond traditional medical facilities. This expansion has profound implications for addressing healthcare disparities and improving outcomes for underserved populations.
Remote monitoring systems allow patients with chronic conditions to remain at home while still receiving close medical supervision. AI algorithms can analyze data from in-home sensors and patient-reported outcomes to detect deterioration before it becomes critical, potentially preventing hospitalizations and emergency department visits.
For rural and underserved communities, AI-augmented telehealth can provide access to specialist expertise that would otherwise be unavailable. As one researcher noted, "AI has the capability of remotely diagnosing patients, thus extending medical services to remote areas, beyond the major urban centers of the world."
The future of connected care isn't just about technology—it's about creating a more patient-centered healthcare system that meets people where they are. By 2030, we can expect to see healthcare delivery models that seamlessly integrate in-person care, virtual visits, remote monitoring, and AI-driven decision support into a cohesive experience tailored to individual patient needs and preferences.
Challenges and Limitations
Regulatory Hurdles and Implementation Barriers
Despite the tremendous potential of AI in healthcare, significant challenges must be overcome before widespread adoption becomes reality. The greatest challenge isn't whether the technologies will be capable enough to be useful, but rather ensuring their adoption in daily clinical practice.
For widespread implementation to occur, AI systems must clear several hurdles. They need regulatory approval from bodies like the FDA and European Medicines Agency, which are still developing frameworks for evaluating AI-based medical technologies. These systems must also be integrated with existing electronic health record (EHR) systems, standardized to ensure interoperability, and taught to clinicians who may have varying levels of technological literacy.
As noted in Future Healthcare Journal, "These challenges will ultimately be overcome, but they will take much longer to do so than it will take for the technologies themselves to mature." Experts predict that while we'll see limited use of AI in clinical practice within 5 years, more extensive adoption may require a decade or more.
Payment models present another significant barrier. Healthcare organizations and medical practices need clear reimbursement pathways for AI-augmented care to justify the substantial investments required for implementation. Until payers recognize the value of these technologies through appropriate compensation mechanisms, adoption may remain limited to large academic medical centers and well-resourced health systems.
Data Privacy and Ethical Considerations
The power of AI in healthcare derives largely from its ability to analyze vast quantities of patient data—but this same capability raises important privacy and ethical concerns. As healthcare AI systems become more sophisticated and widespread, questions about data ownership, consent, and security will become increasingly pressing.
Patients must trust that their sensitive health information will be protected when used to train and operate AI systems. This requires robust security measures, transparent data governance policies, and clear communication about how patient information is being utilized.
Ethical considerations extend beyond privacy concerns. AI systems trained on historical medical data may inadvertently perpetuate or even amplify existing biases in healthcare delivery. For example, if an algorithm is trained primarily on data from one demographic group, it may perform poorly when applied to patients from different backgrounds. Ensuring that AI tools deliver equitable care across diverse populations will require deliberate efforts to identify and mitigate bias in both training data and algorithmic design.
The Human Element: AI as Assistant, Not Replacement
Perhaps the most important consideration in the evolution of medical AI is understanding its proper role in the healthcare ecosystem. Despite dramatic headlines about AI outperforming human clinicians in specific tasks, the evidence increasingly suggests that AI will augment rather than replace healthcare professionals.
"It also seems increasingly clear that AI systems will not replace human clinicians on a large scale, but rather will augment their efforts to care for patients," notes research in Future Healthcare Journal. Over time, the role of human clinicians may evolve toward tasks and job designs that emphasize uniquely human skills like empathy, persuasion, and big-picture integration.
The most successful implementations of healthcare AI will be those that thoughtfully integrate artificial and human intelligence, leveraging the strengths of each. AI excels at pattern recognition, consistent application of rules, and processing vast amounts of data. Humans bring contextual understanding, ethical judgment, and the interpersonal connection that remains central to the healing process.
As one researcher aptly observed, "Perhaps the only healthcare providers who will lose their jobs over time may be those who refuse to work alongside artificial intelligence." The future of healthcare isn't about choosing between human and artificial intelligence—it's about creating synergistic partnerships that improve outcomes for patients and providers alike.
Conclusion
The Road Ahead: What to Expect by 2030
As we've explored throughout this article, artificial intelligence is poised to transform healthcare in profound ways over the next five years. From revolutionizing medical diagnostics to accelerating drug discovery, enabling precision medicine, and extending care beyond traditional settings, AI promises to make healthcare more accurate, accessible, and personalized than ever before.
By 2030, we can expect to see AI firmly established as an essential component of healthcare delivery. The technologies we've discussed will mature from promising innovations to standard tools in the medical arsenal. Diagnostic AI will become increasingly integrated into clinical workflows, drug discovery will be fundamentally AI-driven, and precision medicine approaches will be commonplace rather than exceptional.
According to research published in ScienceDirect, healthcare will shift "from the traditional one-size-fits-all form of medicine to a preventative, personalised, data-driven disease management model that achieves improved patient outcomes in a more cost-effective delivery system." This transformation won't happen overnight, but the foundations are being laid today, and the pace of change will accelerate dramatically over the next decade.
Preparing for an AI-Augmented Healthcare Future
For healthcare providers, preparing for this AI-augmented future means developing new skills and mindsets. Medical education will increasingly incorporate data science and AI literacy, ensuring that the next generation of clinicians can effectively collaborate with intelligent systems. Existing healthcare professionals will need opportunities for continuing education to adapt to these evolving technologies.
For patients, the AI healthcare revolution offers both opportunities and responsibilities. Greater access to health data and AI-powered insights will enable more active participation in healthcare decisions. However, this also requires digital health literacy and thoughtful consideration of how personal health information is shared and utilized.
For healthcare systems and policymakers, the challenge lies in creating environments where AI can flourish while ensuring patient safety, data privacy, and equitable access. This means developing appropriate regulatory frameworks, payment models that incentivize innovation, and infrastructure that supports secure data sharing and interoperability.
The future of healthcare isn't about choosing between human compassion and technological precision—it's about harnessing the best of both. As Microsoft CEO Satya Nadella observed, "AI is perhaps the most transformational technology of our time, and healthcare is perhaps AI's most pressing application." By embracing this transformation thoughtfully, we have the opportunity to create a healthcare system that is not only more efficient and effective but also more humane and accessible to all.
The next five years will be just the beginning of this journey, but they will set the course for decades of innovation to come. The future of medicine is being rewritten by artificial intelligence, and that future is arriving faster than many of us anticipated. The revolution is already underway—and its potential to improve human health is nothing short of extraordinary.
Tables
Table 1: Timeline of AI Medical Breakthroughs
Timeline | Connected/Augmented Care | Precision Diagnostics | Precision Therapeutics |
---|---|---|---|
Short-term: 0-5 years | Internet of things in healthcare Virtual assistants Augmented telehealth Personalized mental health support |
Precision imaging (e.g., diabetic retinopathy screening) AI-powered cancer detection Automated ECG analysis |
Clinical trial design optimization Early-stage drug discovery Adverse effect prediction |
Medium-term: 5-10 years | Ambient intelligence in healthcare Large-scale adoption of precision imaging |
Advanced multi-modal diagnostic systems Integrated diagnostic platforms |
Co-innovation with technology partners Novel AI systems for precision therapeutics |
Long-term: >10 years | Autonomous virtual health assistants Predictive and anticipatory care Networked care organizations |
Holographic and hybrid imaging Fully autonomous diagnostic systems |
Digital twin treatment simulation Fully personalized medicine AI-designed therapeutic molecules |
Timings are illustrative to widescale adoption of the proposed innovation taking into account challenges, regulatory environment, and use at scale.
Table 2: Comparison of AI vs. Human Diagnostic Accuracy in Various Medical Fields
Medical Field | Diagnostic Task | AI Performance | Human Clinician Performance | Source |
---|---|---|---|---|
Radiology | Breast Cancer Detection | 91% sensitivity for early breast cancer | 74% sensitivity for early breast cancer | Kim et al., Lancet Digital Health (2020) |
Radiology | Mammogram Interpretation | Reduced false positives by 5.7% and false negatives by 9.4% | Baseline comparison | McKinney et al., Nature (2020) |
Dermatology | Skin Cancer Classification | Accuracy comparable to board-certified dermatologists | Baseline comparison | Esteva et al., Nature (2017) |
Ophthalmology | Diabetic Retinopathy Screening | High sensitivity and specificity | Limited by availability of specialists | Research studies in USA, Singapore, Thailand, and India |
Cardiology | Heart Attack Diagnosis | Performance comparable to cardiologists | Baseline comparison | Multiple studies |
Respiratory Medicine | Pneumonia Detection | 96% sensitivity, 64% specificity | 50% sensitivity, 73% specificity | Becker et al., Diagnostics (2022) |
Note: Performance metrics vary by study and specific implementation. This table represents findings from published research as cited in the references section.